Po-Hao Chen, MD MBA
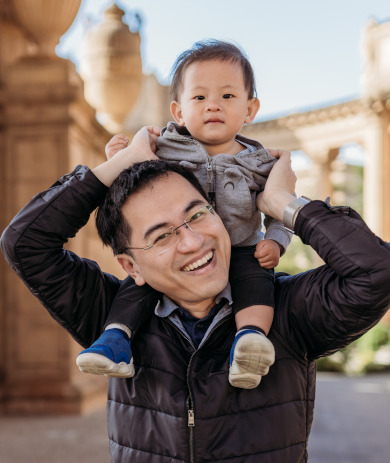
About
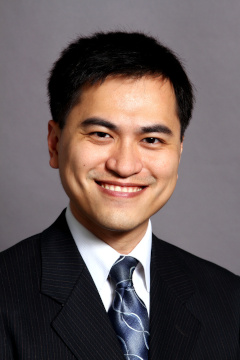
I have passion for translating cutting-edge technology into everyday patient care. At the heart of my work is a commitment to leveraging artificial intelligence and data engineering to make diagnostic services more available, accessible, and affordable.
In my current roles as Vice Chair of Artificial Intelligence for Diagnostics Institute and Medical Director for Enterprise Radiology Informatics at Cleveland Clinic, we built the Center for Intelligent Diagnostics, focusing on the translational application AI to revolutionize diagnostics and treatment planning. In my previous role as the Chief Informatics Officer for Imaging, Cleveland Clinic modernized IT systems for four new hospital acquisitions and replaced radiology PACS for the enterprise's 4 million imaging exams yearly.
Volunteering in leadership roles across key organizations has been among my most fulfilling experiences raising awareness to these real-world technology adoption challenges at a national level. Working with experts in ACR, we championed the responsible adoption of AI through the efforts of Informatics Advisory Council, Commission on Informatics, and through the annual Data Science Summit. Leading SIIM's Security Subcommittee, the team and I tackle healthcare's cybersecurity intersections. At the RSNA, I chair the Informatics Policy Committee, participate in the Government Relations Committee, and act as RSNA's representative to AMA's Specialty Collaboration on AI. As SPIE's Healthcare Informatics Program Committee past chair, I am proud of highlighting new research developments in machine learning. These positions have fueled my growth and impact in the field.
My research endeavors have culminated in authorship in over 70 publications in prestigious journals, abstracts, presentations, posters, and book chapters, across imaging informatics, AI, genomics, and beyond. My innovations have been recognized with two USPTO patents in AI algorithm in CT imaging.
You can find my profile on Twitter, LinkedIn, or follow the blog Figure Stuff Out. I enjoy imaging informatics, healthcare innovation, and good comfort food. The content of this website and the blog are of my personal opinion and do not represent the view of Cleveland Clinic.
Research Projects
Natural Language Processing for Imaging Protocols
AI for Acute Aortic Syndrome Detection
Integrated Workflow Peer Learning Case Submission
ARIES - Deep Learning + Bayesian System for Radiology Education and Dx Support
RadCare - Resident-Led Radiology Consultation for General Medicine Wards
Capricorn - Radiology Residency Analytics Tool
Centaur - Rapid-Fire Learning Module for Basic Imaging Findings
Integrating natural language processing and machine learning algorithms to categorize oncologic response in radiology reports
Unsupervised genomic and epigenetic analysis
Select Publications
- Aleixo GFP, Valente SA, Wei W, Chen P-H, Moore HCF. Sarcopenia detected with bioelectrical impedance versus CT scan and chemotherapy tolerance in patients with early breast cancer. Breast Cancer. 2023 Jan;30(1):101–9.
- Shah C, Chen P-H. Beyond the AJR: Robust Ability of Artificial Intelligence to Detect Race Underscores the Need for Inclusivity and Transparency. Am J Roentgenol. 2022 Dec 21;1.
- Mirzai S, Eck BL, Chen P-H, Estep JD, Tang WHW. Current Approach to the Diagnosis of Sarcopenia in Heart Failure: A Narrative Review on the Role of Clinical and Imaging Assessments. Circ Heart Fail. 2022 Oct;15(10):e009322.
- Xavier BA, Chen P-H. Natural Language Processing for Imaging Protocol Assignment: Machine Learning for Multiclass Classification of Abdominal CT Protocols Using Indication Text Data. J Digit Imaging. 2022 Jun;35:1120-30.
- Chen P-H, Bodak R, Gandhi NS. Ransomware Recovery and Imaging Operations: Lessons Learned and Planning Considerations. J Digit Imaging. 2021 Jun;34(3):731-740.
- Rudie JD, Duda J, Duong MT, Chen P-H, Xie L, Kurtz R, et al. Brain MRI Deep Learning and Bayesian Inference System Augments Radiology Resident Performance. J Digit Imaging.s 2021 Aug;34(4):104958.
- Shah C, Kohlmyer S, Hunter K, Jones SE, Chen P-H. A translational clinical assessment workflow for the validation of external artificial intelligence models. In: Park BJ, Deserno TM, editors. Medical Imaging 2021: Imaging Informatics for Healthcare, Research, and Applications. Online Only, United States: SPIE; 2021. p. 14.
- Martin-Carreras TT, Li H, Chen P-H. Interpretative applications of artificial intelligence in musculoskeletal imaging: concepts, current practice, and future directions. J Med Artif Intell. 2020 Sep;3:1313.
- Shah C, Cook TS, Chen P-H, Hyland S, Heavener R, Kahn CE, et al. Improving Triage of After-Hours Radiology Examinations Through Worklist Unification. J Am Coll Radiol. 2020 Mar;S1546144020301459.
- Martin-Carreras T, Chen P-H. From Data to Value: How Artificial Intelligence Augments the Radiology Business to Create Value. Sem Musc Radiol. 2020 Feb;24(01):6573.
- Chen P-H. Essential Elements of Natural Language Processing: What the Radiologist Should Know. Acad Radiol. 2020 Jan;27(1):612.
- Duong MT, Rauschecker AM, Rudie JD, Chen P-H, Cook TS, Bryan RN, et al. Artificial intelligence for precision education in radiology. Br J Radiol. 2019 Jul 26;20190389.
- Gillman J, Wu SE, Rowland J, Scanlon M, Chen P-H. Comparison of In-Person and Digital Radiology Resident Consultation Services. J Am Coll Radiol. 2019 Feb 4; S1546144018314856
- Chen P-H, Scanlon MH. Teaching Radiology Trainees from the Perspective of a Millennial. Academic Radiology. 2018 Jun;25(6):794-800.
- Chen P-H, Cross N. IoT in Radiology: Using Raspberry Pi to Automatically Log Telephone Calls in the Reading Room. Journal of Digital Imaging. 2018 Jun;31(3):371-8.
- Deitte LA, Chen P-H, Scanlon MH, Heitkamp DE, Davis LP, Urban S, et al. Twenty-four-Seven In-house Faculty and Resident Education. Journal of the American College of Radiology. 2018 Jan;15(1):90a.
- Chen P-H, Zafar H, Galperin-Aizenberg M, Cook T. Integrating Natural Language Processing and Machine Learning Algorithms to Categorize Oncologic Response in Radiology Reports. J Digit Imaging. 2018 Apr;31(2):17884.